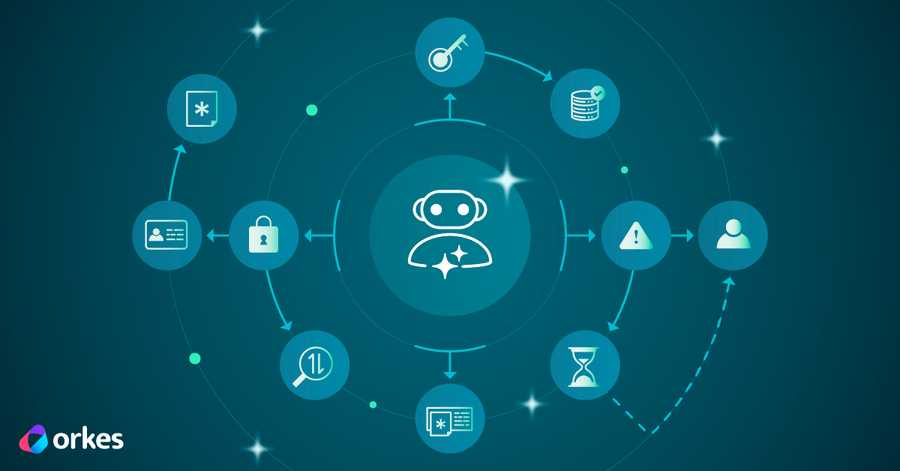
ALL, AGENTIC, SOLUTIONS
Tame Your Agent: Build a Secure, Contained Customer Success AI Agent
June 17, 2025
Got AI Trust Issues? Me too. So let’s build an AI agent that’s powerful and responsible in this visual step-by-step guide....
Orchestration coordinates services, agents, and humans into automated flows, reducing fragmentation, tech debt, and operational inefficiencies....
All
Agentic
Product
Engineering
Solutions
Community
Announcements
Insights
ALL, AGENTIC, SOLUTIONS
June 17, 2025
Got AI Trust Issues? Me too. So let’s build an AI agent that’s powerful and responsible in this visual step-by-step guide....
ALL, ANNOUNCEMENTS
June 09, 2025
Announcing the Conductor MCP Server — a lightweight API layer that gives AI agents real-time control over Conductor workflows....
ALL, SOLUTIONS
May 29, 2025
SerDe tests ensure that data objects are correctly converted for interoperability. Learn how to automate test code creation using orchestration and AI...
ALL, COMMUNITY
May 26, 2025
A packed night of Mastering AI Agents and Agentic Workflows at SPIN Seattle. Top takeaways, fun moments, and a glimpse at what’s ahead with Orkes Cond...
ALL, AGENTIC, INSIGHTS
May 26, 2025
What is Model Context Protocol (MCP)? Learn how MCP simplifies AI integration by enabling seamless connections between agents and third-party tools...
ALL, INSIGHTS
May 22, 2025
Discover more about orchestration, a powerful alternative to BPM tools for designing and automating business processes at scale....
ALL, AGENTIC, INSIGHTS
May 19, 2025
Agentic AI systems can be implemented as agents or as workflows. Learn how they are different and which strategy to use....
ALL, PRODUCT
May 12, 2025
Learn how to use timeouts, retries, and failure workflows in Orkes Conductor to build resilient, self-healing distributed systems....
ALL, PRODUCT
May 12, 2025
Learn how to use workflow timeouts and failure workflows in Orkes Conductor to build resilient, SLA-compliant orchestrations that recover gracefully f...
ALL, PRODUCT
May 12, 2025
Learn how to use task retries, timeouts, and system-level safeguards in Orkes Conductor to build resilient, fault-tolerant workflows....
ALL, ANNOUNCEMENTS, PRODUCT
May 09, 2025
Instantly convert legacy BPMN files into modern workflows with the new BPMN Importer in Orkes Conductor. No code, no rewrites—just fast, accurate orch...
ALL, PRODUCT
April 28, 2025
Learn the importance of using control flow operators to declaratively build dynamic, responsive workflows without plumbing code....
ALL, PRODUCT
April 28, 2025
Master branching and conditional flows in Orkes Conductor workflows using operators....
ALL, PRODUCT
April 28, 2025
Add loops, waits, and human approvals to Orkes Conductor workflows with simple, structured operators....
ALL, PRODUCT
April 28, 2025
Discover how to scale workflows in Orkes Conductor with Fork/Join, Dynamic Fork, SubWorkflow, and StartWorkflow operators for parallelism and reusabil...
ALL, SOLUTIONS
April 24, 2025
Solve manual claims processing inefficiencies with AI automation and orchestration. Get an in-depth technical breakdown for building with Conductor....
ALL, AGENTIC, SOLUTIONS
April 21, 2025
Learn how to build a fully automated technical interview workflow using Orkes Conductor. This step-by-step guide walks you through orchestrating LLM-p...
ALL, PRODUCT
April 14, 2025
Learn how to use built-in system tasks in Orkes Conductor for real-life use cases....
ALL, PRODUCT
April 14, 2025
Use built-in system tasks to accelerate development time, enable low-latency execution, and ensure enterprise-grade availability in Conductor....
ALL, AGENTIC, SOLUTIONS
April 09, 2025
Use agentic workflows to add AI-driven dynamism in a governable and traceable manner. Try it with Orkes Conductor....
ALL, ENGINEERING
April 04, 2025
Learn how to orchestrate long-running APIs using Orkes Conductor, AWS Lambda, API Gateway, and DynamoDB with asynchronous invocation and polling....
ALL, AGENTIC, INSIGHTS
April 01, 2025
Agentic workflows are an AI-driven processed where the sequence of tasks are dynamically executed with minimal human intervention to achieve a particu...
ALL, ENGINEERING
March 26, 2025
Using asynchronous workflows enables responsive and resilient systems even in the face of uncertainty....
ALL, AGENTIC, INSIGHTS
March 18, 2025
Find out how agentic AI, combined with BOAT tools, will accelerate the world of business automation into the realm of intelligent, adaptive decision-m...
ALL, SOLUTIONS
March 17, 2025
Learn how to automate email nurturing workflows with SendGrid webhooks and Orkes Conductor for improved lead conversion and personalized follow-ups....
ALL, INSIGHTS
March 04, 2025
Using BOAT software, implement the ten best practices that will help you master business process orchestration....
ALL, SOLUTIONS
February 25, 2025
Discover why service uptime monitoring is crucial for businesses. Learn how Orkes Conductor helps ensure 99.999% uptime and prevent downtime with auto...
ALL, ENGINEERING
February 20, 2025
Learn hands-on the best practices to improve RAG accuracy and response quality: contextual headers, semantic chunks, hybrid search, and reranking....
ALL, SOLUTIONS
February 05, 2025
Learn how to build an AI document classification app using Orkes Conductor and LLMs to automate and streamline document sorting and improve efficiency...
ALL, INSIGHTS
January 21, 2025
The AI trends businesses should watch for in 2025: 1. AI redefining user-computer interactions 2. Evolving AI capabilities 3. Agentic AI to mature 4. ...
ALL, COMMUNITY
January 16, 2025
Conductor OSS has a brand new visualizer for its workflow definition and execution diagrams....
ALL, ENGINEERING
January 07, 2025
Learn about realtime API orchestration and other key strategies to reduce latency, streamline API workflows, and optimize application performance....
ALL, ENGINEERING
November 27, 2024
Experiment with more effective LLM prompts by leveraging model choice, prompt engineering tactics, and parameter tuning....
ALL, ENGINEERING
November 21, 2024
Get a guided tutorial on how to develop an AI application with minimal technical overhead using orchestration....
ALL, ENGINEERING
November 04, 2024
The Backend for Frontend (BFF) pattern customizes backend services for each frontend. Learn how to implement a BFF layer with Orkes Conductor....
ALL, ANNOUNCEMENTS
October 25, 2024
Candid Conversations is a new video series exploring trends in workflow orchestration, AI, and tech’s impact on modern app development....
ALL, ENGINEERING
October 23, 2024
Change Data Capture (CDC) is a technique for identifying and capturing changes in a database and replicating them in real time to other systems....
ALL, ANNOUNCEMENTS
October 14, 2024
Orkes is thrilled to announce Java Client v4, featuring significant design enhancements, performance improvements, and optimized dependencies....
ALL, ENGINEERING
October 03, 2024
Figure out if your monolith should be split into microservices and learn the best practices for planning, executing, and testing your migration projec...
ALL, ENGINEERING
September 09, 2024
Debugging distributed systems is tricky due to observability or reproducibility issues. A key strategy lies in using tools that cut out time to track ...
ALL, INSIGHTS
August 23, 2024
Orchestration is the process of coordinating distributed software components and systems so that they execute seamlessly as an automated, repeatable p...
ALL, ENGINEERING
August 06, 2024
Software architecture has evolved from mainframes and monoliths into a distributed network of cloud computing, API connectivity, AI, and microservices...
ALL, ANNOUNCEMENTS
July 22, 2024
Orkes is officially part of Singapore's IMDA Spark Programme as of March 2024, allowing Orkes to facilitate deeper engagement with the local tech comm...
ALL, INSIGHTS
July 15, 2024
In AI orchestration, a central platform coordinates interactions between AI components. Discover the different ways to use AI in your business applica...
ALL, ANNOUNCEMENTS
July 02, 2024
Orkes Conductor offers integrations with Amazon Bedrock, providing greater flexibility for orchestrating workflows using AI....
ALL, ENGINEERING
June 27, 2024
Learn the fundamentals of prompt engineering, what it is, its significance in app development, and how to build LLM-powered applications effectively....
ALL, ENGINEERING
June 13, 2024
RAG (retrieval-augmented generation) improves LLM output with pre-fetched data from external sources, enabling developers to build semantic search fea...
ALL, ANNOUNCEMENTS
June 05, 2024
A new Enterprise Base plan with a 14-day free trial of Orkes Conductor. Users can sign up and immediately get access to an Orkes Conductor cluster....
ALL, PRODUCT
May 28, 2024
Conductor is an orchestration engine for creating complex flows across distributed components. Learn the differences between OSS and Orkes....
ALL, INSIGHTS
May 17, 2024
Dive into the latest developments in AI, API, and automation and discover how to leverage them for your business....
ALL, COMMUNITY
May 13, 2024
Explore the highlights from the AI Orchestration Meetup in Bengaluru, featuring insights on GenAI, AI orchestration, and implementation using Orkes Co...
ALL, ENGINEERING
May 09, 2024
Learn more about the technical implementation of durable systems and how Conductor delivers resilience with its decoupled infrastructure and redundanc...
ALL, INSIGHTS
May 08, 2024
Durable execution enables applications to continue running in face of failure. Find out how to implement it, especially for long-running processes....
ALL, SOLUTIONS
April 17, 2024
Learn how to use Conductor-Webhook integration to automate slack greetings to new community members....
ALL, SOLUTIONS
April 08, 2024
Find out how to upgrade EKS clusters using Conductor, with in-built failure handling, scheduling, and more....
ALL, ANNOUNCEMENTS
February 21, 2024
Orkes’ Series A funding will power the next phase of growth for Conductor as the development platform of choice....
ALL, ANNOUNCEMENTS
February 09, 2024
Conductor’s Python SDK has been refactored, providing support for AI orchestration, a better experience for creating dynamic workflow, and more....
ALL, COMMUNITY
January 31, 2024
Learn more about the happenings from Orkes in Jan 2024....
ALL, ANNOUNCEMENTS
January 21, 2024
Announcing the initial release of Conductor OSS from Orkes....
ALL, COMMUNITY
December 22, 2023
Check out the latest December 2023 updates in Orkes....
ALL, SOLUTIONS
December 21, 2023
Learn how to integrate your Conductor orchestration stack with Opsgenie to get incident alerts and swiftly take action....
ALL, COMMUNITY
December 15, 2023
Learn from our community on how to Conductor OSS to set up a Digital Public Goods (DPG) compliant orchestration layer in your applications....
ALL, COMMUNITY
December 13, 2023
Orkes will take stewardship of Conductor OSS in collaboration with the community and will remain committed to a developer-first experience....
ALL, COMMUNITY
November 30, 2023
Check out the latest November 2023 updates in Orkes....
ALL, ANNOUNCEMENTS, PRODUCT
November 02, 2023
Seamlessly integrate LLM capabilities and vector databases into applications using AI orchestration and native prompt engineering support....
ALL, COMMUNITY
October 31, 2023
Check out the latest October 2023 updates in Orkes....
ALL, ENGINEERING
October 13, 2023
Learn the four most important patterns for microservices: CQRS pattern, strangler pattern, publisher/subscriber pattern, and saga pattern....
ALL, COMMUNITY
October 03, 2023
Check out the latest September 2023 updates in Orkes....
ALL, SOLUTIONS
September 22, 2023
Automate wildcard certificate deployment at scale with Conductor so that cluster access is always kept secure....
ALL, COMMUNITY
August 31, 2023
Check out the latest August 2023 updates in Orkes....
ALL, PRODUCT
August 10, 2023
Learn how to control a running workflow by sending signals to the workflow execution....
ALL, ENGINEERING
August 01, 2023
Learn how to use tools like Prometheus and Grafana for monitoring Conductor workflows....
ALL, COMMUNITY
July 31, 2023
Check out the latest July 2023 updates in Orkes....
ALL, SOLUTIONS
July 24, 2023
Find out how to implement a saga pattern in your applications using Orkes Conductor, an orchestration tool....
ALL, ENGINEERING
July 14, 2023
Discover the best tools, languages, and frameworks for accelerating the development cycle, from API testing to performance monitoring....
ALL, SOLUTIONS
July 07, 2023
Learn how to create a Slack bot that sends reminders to post daily stand-up messages using Conductor-Webhook integration...
ALL, COMMUNITY
June 30, 2023
Check out the latest June 2023 updates in Orkes....
ALL, COMMUNITY
June 09, 2023
Get Orkes’ recap of the highlights from the 2023 Microsoft Build conference and Gartner Application Innovation & Business Solutions Summit....
ALL, SOLUTIONS
June 01, 2023
Learn how to build a subscription management system with Spring Boot and Orkes Conductor....
ALL, COMMUNITY
June 01, 2023
Check out the latest May 2023 updates in Orkes....
ALL, COMMUNITY
May 31, 2023
Get a recap of the highlights from Orkes Bangalore meetup in April 2023....
ALL, PRODUCT
May 31, 2023
Discover how to use webhooks in Conductor workflow for a variety of use cases in microservice communications....
ALL, ENGINEERING
May 22, 2023
Distributed transactions can safely recover from failures using rollback mechanisms like the saga pattern....
ALL, SOLUTIONS
May 19, 2023
Use Conductor, a workflow orchestration platform, to automate cloud infrastructure upgrades with no downtime....
ALL, COMMUNITY
May 03, 2023
Check out the latest April 2023 updates in Orkes....
ALL, PRODUCT
April 28, 2023
Use Conductor to transition from a monolithic to a microservice-based architecture and to modernize your applications....
ALL, ENGINEERING
April 26, 2023
Transactional backends can be implemented using a database-oriented approach or a workflow-oriented approach....
ALL, PRODUCT
April 13, 2023
Discover more about Conductor’s Scheduler feature and automate your workflows at custom intervals....
ALL, COMMUNITY
April 04, 2023
Check out the latest March 2023 updates in Orkes....
ALL, ENGINEERING
March 24, 2023
Learn the differences between orchestration and choreography, and the advantages and drawbacks of each approach....
ALL, ENGINEERING
March 14, 2023
Conductor is a workflow engine, which can help automate routine tasks, enable faster application deployment, and more....
ALL, PRODUCT
March 03, 2023
Writing workflows with code unlocks greater flexibility in creating dynamic workflows on the go. Try out Conductor’s SDKs today....
ALL, COMMUNITY
March 01, 2023
Check out the latest February 2023 updates in Orkes....
ALL, PRODUCT
February 24, 2023
Discover how to use LLMs, like ChatGPT, as a coding co-pilot to generate Conductor workflows as code....
ALL, PRODUCT
February 14, 2023
Learn how to automate a long-running user subscription workflow using Conductor, an orchestration platform....
ALL, COMMUNITY
January 31, 2023
Check out the latest January 2023 updates in Orkes....
ALL, SOLUTIONS
January 13, 2023
Learn how to build a checkout application using Next.js and a workflow as code approach in Conductor....
ALL, PRODUCT
January 10, 2023
Based on benchmark tests, Conductor’s workload performance demonstrates consistent throughput across task execution, data processing, and more....
ALL, PRODUCT
January 06, 2023
Use Conductor to build transaction dispute workflows and get native support for retries, scaling, debugging, and more....
ALL, COMMUNITY
January 02, 2023
Explore Orkes updates and highlights from December 2022....
ALL, COMMUNITY
December 21, 2022
Get the key highlights from Orkes's participation at DevFests in 2022....
ALL, COMMUNITY
November 29, 2022
Check out the latest November 2022 updates in Orkes....
ALL, COMMUNITY
November 25, 2022
Orkes’ first hackathon, the Orkes Hack, was held online and participants competed to create the most creative workflow in Conductor....
ALL, ANNOUNCEMENTS
November 18, 2022
Orkes has attained SOC 2 Type 2 compliance in our continued endeavor to provide the highest standards in data security for Orkes Cloud....
ALL, ANNOUNCEMENTS, PRODUCT
November 17, 2022
Orkes Conductor has webhook integrations available for seamless integration with third-party applications....
ALL, SOLUTIONS
November 10, 2022
Learn how to build a food delivery application using TypeScript and a workflow as code approach in Conductor....
ALL, PRODUCT
August 09, 2022
Orkes Conductor supports the use of secrets to securely send sensitive parameters in workflows....
ALL, SOLUTIONS
August 05, 2022
Discover how to implement KYC (Know Your Customer) checks for high-value transactions using Conductor....
ALL, PRODUCT
August 05, 2022
Learn how to reuse tasks and workflows in Conductor, with reusable tasks, sub workflows, dynamic tasks, do/while operators, and more....
ALL, ENGINEERING
July 05, 2022
Orchestration is a centralized approach to coordinating microservices, with a clear view of the overall process, while choreography is more decentrali...
ALL, ENGINEERING
June 09, 2022
Learn how to break up a monolithic application into microservice-based architecture for greater scalability and ease of deployment....
ALL, ENGINEERING
June 01, 2022
Monolithic architecture creates a tightly coupled codebase that takes longer to update and deploy, which can be resolved by breaking it up into micros...
ALL, ENGINEERING
May 25, 2022
Using multiple languages in a microservice architecture provides greater flexibility, but may require more maintenance....
ALL, SOLUTIONS
May 12, 2022
Learn how to build a Conductor workflow for data processing, which follows the three-phase ETL (extract, transform, load) process....
ALL, ANNOUNCEMENTS
May 03, 2022
Announcing the new open-source repository for Orkes Conductor....
ALL, ENGINEERING
May 03, 2022
Polyglot microservice orchestration enables developers to use multiple programming languages for various purposes in a single workflow....
ALL, PRODUCT
April 21, 2022
Try using Conductor to run tasks and workers in Clojure....
ALL, PRODUCT
April 18, 2022
Workflows are like recipes: a series of tasks that must be completed in order, with varying degrees of complexity....
ALL, ENGINEERING
April 13, 2022
Learn the benefits and limitations of a microservice architecture, with an example use case in virtual goods....
ALL, ENGINEERING
April 06, 2022
Hybrid cloud architecture makes it easier to manage, scale, and migrate your infrastructure while reducing operation costs....
ALL, ANNOUNCEMENTS
March 30, 2022
Orkes has launched new Conductor SDKs for C# and Clojure and made major improvements to Golang and Python SDKs....
ALL, ENGINEERING
March 24, 2022
Microservice orchestration helps teams to build and scale applications swiftly without creating a distributed monolith....
ALL, ENGINEERING
March 21, 2022
Conductor is an orchestration platform that connects distributed microservices so data flows without needing to build additional infrastructure....
ALL, ANNOUNCEMENTS
March 08, 2022
Announcing Conductor Playground, a full-featured sandbox environment to try Orkes Conductor for free....
ALL, SOLUTIONS
March 03, 2022
See workflow orchestration in action with Conductor, using a loan banking workflow example....
ALL, ANNOUNCEMENTS
February 28, 2022
Announcing Orkes Cloud, a hosting solution that manages your Conductor deployment and infrastructure for you....
ALL, COMMUNITY
February 10, 2022
Get a recap of Orkes’ Feb 2022 developer meetup, where invited speakers share how they use Conductor in production at their companies....
ALL, SOLUTIONS
February 02, 2022
Learn how to modularize and re-use workflows in Conductor using sub workflows....
ALL, SOLUTIONS
January 31, 2022
Learn how to use Conductor’s dynamic forks, which creates the appropriate number of parallel processes at runtime....
ALL, SOLUTIONS
January 31, 2022
Learn how to use Conductor to build an image processing workflow that can automatically create multiple sizes and formats for the same image....
ALL, PRODUCT
January 25, 2022
Learn how Conductor handles retries and timeouts with its built-in error-handling functionalities....
ALL, SOLUTIONS
January 24, 2022
Learn how to use Conductor to build an image processing workflow that can automatically complete tasks like resizing or format conversion....
ALL, ENGINEERING
December 15, 2021
Orchestration provides a systematic approach to coordinating microservices and offers more visibility into process flows compared to choreography....
ALL, INSIGHTS
November 16, 2021
Find out how Conductor got its start as an open-source orchestration engine, originally built by Netflix to solve infrastructure issues in microservic...